December 2023
Pupdate
I already wrote about Milo’s lymphoma going into remission, which is good :)
We’re probably hitting more setbacks due to low neutrophils than last time around, but the whole process has (sadly) become routine, so it’s all less emotional and dramatic.
Despite a succession of named storms we managed to get in some good long walks.
Full Fibre Internet
I already wrote about the saga of getting it installed, but after over a week of use I’m very pleased with the service. Download speeds are ridiculously quick now for some stuff. Latency and jitter also seem to be improved, which gives an overall impression of better quality.
Solar Diary
This December was much cloudier than last year :(
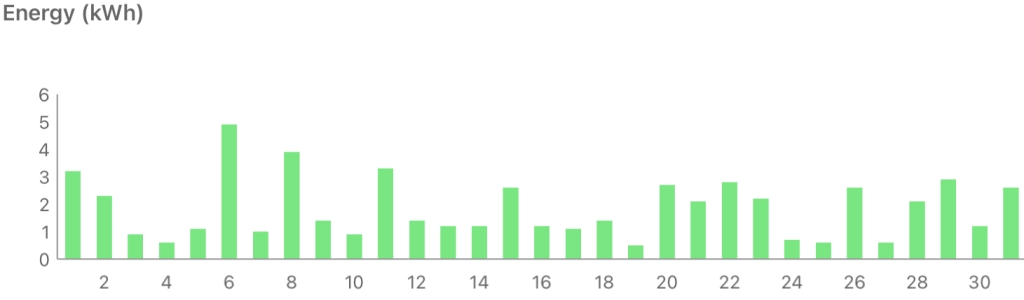
Beating Beat Saber
I played a little Beat Saber at the start of the month. Despite controller updates the tracking still isn’t perfect, but it’s certainly improved.
The new VI levels are a lot of fun :)
But… I’ve been trying some other Quest games:
Gun Club VR
I got an email saying there was £5.70 of credit on my Oculus account so I took a look at the store to see what that would buy me. Gun Club VR was one of the many games in the holiday sale, and the £2.29 it cost me might be the best value I’ve ever found in gaming.
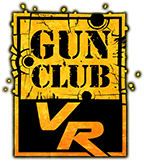
It’s provided many hours of entertainment so far. The only thing that’s more fun than shooting zombies is shooting Nazi zombies :)
I can see myself buying some DLC levels if only to unlock the AR15.
Asgard’s Wrath 2
I didn’t play the original, as it was a PC VR title, but this was bundled with the Quest 3, and released this month.
I’ve only played just past the intro scenes, but so far it’s very impressive and shows high production values and good gameplay.
Fruit Ninja 2
I didn’t bother with the original as I’d played the iPad version and it seemed like too much overlap with Beat Saber.
The new version adds archery, and after my 15m of free trial it was an easy choice to hit the buy button.
Filed under: monthly_update | Leave a Comment
Tags: Asgard's Wrath 2, broadband, Fruit Ninja 2, FTTP, full fibre, games, Gun Club, pupdate, Quest 3, remission, solar, vr
Full Fibre Saga
Almost two weeks later than planned, and after almost three days without an Internet connection to the house it seems like I finally have a working Fibre To The Premises (FTTP) install. Phew! This is the story of how it took so long, and the regulatory failure that underpins the sorry state of affairs.
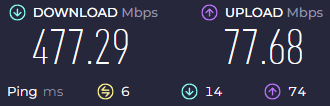
Background
My house was built in 2002. It’s utterly ridiculous that I didn’t get FTTP when I moved in, but as former British Telecom (BT) Chief Technology Officer Dr Peter Cochrane recounts in “How Thatcher killed the UK’s superfast broadband before it even existed” there’s an explanation for why FTTP wasn’t a thing in the UK 21 years ago.
At the time the best on offer was Asynchronous Digital Subscriber Line (ADSL), a service based on tech that BT originally developed to deliver on demand TV services over it’s copper telephone network. That got me 2Mbps, and it wasn’t long before ADSL2 came along and the speed bumped up to 8Mbps[1].
A decade or so rolled by, and along came Fibre To The Cabinet (FTTC) services based on Very-high-speed Digital Subscriber Line (VDSL). I switched to Plusnet (which is a BT subsidiary) as my provider, and they pretty reliably delivered 80Mbps download (~12Mbps up). This has been good enough for the 10+ years I’ve been using it, and when ‘full fibre’ services started to roll out I didn’t feel much pressure to upgrade, especially given the premium.
That cost equation changed though. When my contract came around for renewal this year the VDSL service was priced higher than FTTP, so it was time to change, even if that meant sacrificing the landline[2].
How things should work
The critical piece of equipment for a full fibre install is an Optical Network Termination (ONT). It’s essentially a type of Modulator/Demulator (Modem) taking flashing light from the fibre and turning it into electrical signals on an Ethernet cable that goes into a router (and turning electrical signals coming from the router into flashing light back down the fibre).
The ONT is connected to a Connectorised Block Terminal (CBT) out in the street, which makes use of a form of encryption key based multiplexing so that the fibre from many houses can be back hauled on a single fibre to the exchange.
To connect the ONT to the CBT an engineer needs to run some fibre from the ONT (inside the house) to a junction box (outside the house) and from that junction box to the CBT. In my case there was ducting for both cable runs (holding the copper telephone cable used by existing services), so it should have been very straightforward:
- Fish a couple of fibre optic cables
- Splice those cables together in the junction box
- Activate the new service[3]
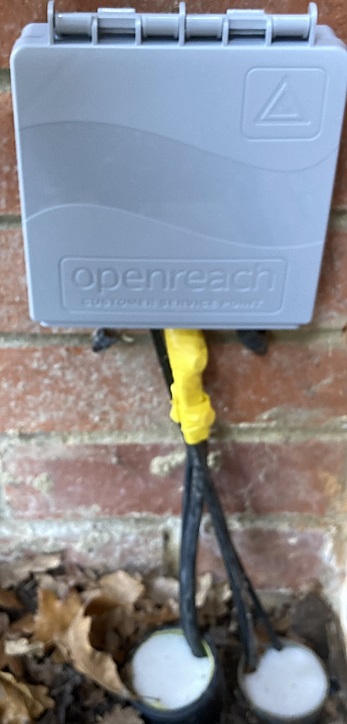
“What’s Involved in an Openreach FTTP Full-Fibre Broadband Installation?” provides a much more detailed description of the process.
Engineer Visit 1 – 8 Dec – Matthew
When I was placing my upgrade order it was implied that installation would happen in a few days, but when it came to selecting a date, the earliest on offer was more than 3 weeks away – Dec 8.
When the day came around Matthew from Kelly Communications (an OpenReach contractor) arrived and set about doing both cable runs. He achieved neither. The internal duct was blocked, though he could just drill a hole through the wall to get around that. But also he couldn’t reach the CBT outside – his ‘cobra’ duct rod wasn’t getting through to the pit. He’d have to escalate the job to a specialist team with the equipment and skills to get past the block.
Clearing the internal duct
My colleague Colin (a former BT engineer) mentioned that he’d seen installers using compressed air to get cables through ducting so I gave that a go. To no avail – there was definitely a blockage. So I grabbed the vacuum cleaner and sucked out some debris (mostly bits of plaster) that likely found their way down their during the house build.
I was then able to get my metal fish line through the duct and leave a piece of string ready.
Engineers, but not for me
On Dec 14 I heard some engineers working outside, but my joy/hope was quickly quashed when they made clear they were working on my next door neighbour’s connection and didn’t have a job card to help me. I called Plusnet to find out when I could expect a visit, but didn’t get any clear answer.
Price hike
The next day (15 Dec) brought a fresh bill for my VDSL service, and as I’d run past the end of the 1yr contracted discount the price had leapt from £28.59 to £54.39. I called Plusnet customer service, and they were pretty swift in applying a £25.80 discount. I guess these things happen a LOT.
Engineer Visit 2 – 18 Dec – Josh
Josh arrived without any warning (having driven all the way from Kent), and was friendly and eager to get on with things. He quickly confirmed that he too couldn’t get from my house to the CBT, and went off to get a camera from a colleague to help navigate the blockage.
A little while later Josh figured out what was going wrong. The trunk from my house goes to a pit to the left of my front door, not to the right where the CBT is. The cable had to be run to pit (left) before going through another trunk to pit (right) where it could be connected.
Sadly the pull string I’d run broke when Josh tried to use it. But we worked together to fish the internal cable, and it wasn’t long until he had the ONT hooked up.
There was a problem though. Josh had only been given the job to get the cable connected. He wasn’t able to activate the service. Josh left assuring me that another engineer would be along ‘in a few days’ to finish things off, and that my VDSL would remain active until then.
Cut off
About 30m after Josh left my VDSL broadband stopped working. I called Plusnet, was quickly escalated to Michael in tier 2 support, and assured that my new service would be activated in the next few hours.
Overnight I got an email ‘Your broadband product change is now complete’, but the new service wasn’t working.
I called Plusnet again, and was told that another engineer visit would be needed, and it would be ‘in a few days’. Meanwhile it was suggested that I could use a ‘BT Hotspot’ to get on the Internet, and I was told about the compensation rates for days without service and missed engineer visits. Later that day I got a text:
We have identified an issue which requires an engineer to attend your property. We have booked the appointment for the engineer to arrive between 08 AM and 01 PM on 21/12/2023
Jury rig
I connected a GL.iNet travel router to an EE Hotspot[4] and then used that as the Internet source for my home router. It worked surprisingly well, showing ~10Mbps down and 20Mbps up on a speed test.
The main annoyance was getting through the EE captive portal to pay and then sign in, which had to be repeated each time a session was timed out :(
Engineer Visit 3 – 21 Dec – Mark
Mark arrived shortly after 8 and immediately saw that the ONT hadn’t been activated.
He was unable to activate it himself with the app on his phone, and had to call a help desk in India who took around 15m to manually activate the service; leaving us both to enjoy hold music.
At that stage things were looking good. The PON light was solid green on the ONT and the multi-colour light on the Plusnet ‘Hub 2’ router was blue indicating a connection. I did a quick speed test with my laptop (over WiFi) and ~400Mbps down and 80Mbps up looked pretty respectable.
Mark wasn’t happy with the test results he was seeing though, and eventually set about checking/cleaning all the connectors. After redoing the splice in the wall junction box line levels were within the acceptable range.
I put the Hub 2 back in its box where it belongs and connected the ONT to my Draytek 2866.
How this could have gone better
I’ll pick some specifics from my experience that will lead into some more general points:
- Matthew should have known which pit he was trying to get to.
- The guys doing next door should have been able to grab my job out of the queue and save one of their colleagues another trip.
- Josh should have been tasked with completing the job, not just doing 90% of it.
- The lack of ONT activation should have prevented the changeover from VDSL to FTTP that cut me off.
- ONT activation could have been handled without Mark’s visit.
- Mark shouldn’t have needed to escalate to the team in India.
The Goal
My DevOps friends will probably know where I’m going here…
I asked one of the engineers how often things just work as they should. I expected the answer to be 50-60%. OpenReach have a well deserved reputation for poor service, but surely things work like they should some of the time. His answer shocked me – ‘never’. He said he’d never seen a ‘textbook’ installation.
The disaster that unfolded over the past weeks bears many of the same hallmarks as my last encounter with OpenReach. As an organisation it seems that their processes and the systems that implement them are fragile, siloed and have poor escalation paths – even for mainstream stuff like FTTP. This is hugely wasteful of precious engineer resource, which leads to customer frustration and poor profitability.
It seems that the regulator OFCOM’s response to this is to allow competition on the ‘last mile’, which should be a natural monopoly. So in addition to OpenReach’s fibre I now have fibre from a bunch of other companies running past my front door. This is a disaster for consumers, as ultimately we’ll all pay for that duplication in higher service charges over the coming years.
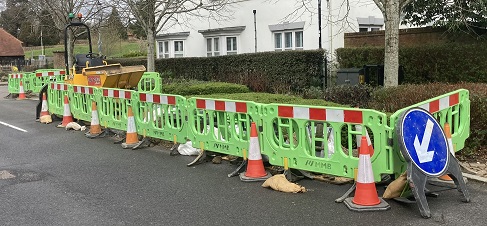
Rather than neoliberal ideology of solving problems with ‘markets’ and ‘competition’ OFCOM should have straightened out OpenReach’s disastrous mismanagement[5]. The problems described above can all be solved:
- by understanding processes end to end
- by empowering staff to do the right thing
- by supporting people with well thought through platforms
- by providing effective means to deal with exceptions when they arise
This is exactly what we did to a bunch of Delivery when I was at CSC/DXC. It’s a hard slog, but worth it. There’s no magic, we simply followed the approach described by Eli Goldratt in The Goal – identify constraints, fix the worst one, repeat.
Updates
22 Dec 2023 – the initial version of this post stated that wavelength division multiplexing (WDM) was used between the ONT and CBT, which has been corrected.
Notes
[1] At around the same time my brother in law was getting his flat in Japan connected to the Internet. His service was 1Gbps symmetric full fibre, and cheaper than my ADSL :0
[2] Plusnet don’t offer the ability to transfer a landline number to a Voice Over Internet Protocol (VOIP) service (unlike BT’s consumer service). In theory I could have picked another service, and hopefully even kept my (easy to remember) ‘home’ number. But even at a few pounds a month it’s hard to justify any cost. I stopped answering that phone many years ago as it was never for me (and usually scammers).
[3] As far as I can tell the main purpose of activation is to bind the ONT encryption key to the upstream Optical Line Terminal (OLT). This is just an entry in a database somewhere. Details of this are pretty sketchy, and I suspect that’s because there’s a bunch of security by obscurity going on. The Wikipedia article on Passive optical network provides the best overview I can find.
[4] It seems the BT Hoptspots have been re-branded to EE (and nobody’s upgraded the Plusnet support scripts).
[5] I should make clear that all of the engineers I encountered were all great – professional, understanding, friendly, and as accommodating as they could be within the constraints inflicted on them. But they work for an organisation that management have allowed to be dysfunctional.
Filed under: could_do_better, technology | Leave a Comment
Tags: BT, adsl, fibre, PlusNet, OpenReach, full fibre, FTTP, VDSL, OFCOM, ONT, CBT, FTTC
A few milestones have ticked by since the last update:
- It’s now over a year since Milo first got ill
- We’ve made it through the first round of his second chemo CHOP protocol
- The scan on the day that should have marked the end of that first round showed ‘a resolution of the intestinal mass and a reduction in size of the enlarged abdominal lymph nodes’
- The oncologist used the word ‘remission’ (even if it can’t be found in the report sent to my local vet)
That’s all good news. Though it comes against a background of some blood tests showing low neutrophil counts, which have led to delays in administering chemo, and more vet visits.
Insurance
ManyPets seem to have slipped back into taking months to process claims. The first claim for this policy was turned around in a couple of days (minus the excess), but everything since then has been queued up. I’m still waiting on a claim for a scan from the end of September. It’s not yet as long a wait as some claims from last year’s policy, but with the holidays almost upon us it’s not looking good.
Past parts:
Filed under: MiloCancerDiary | Leave a Comment
Tags: cancer, chemo, chemotherapy, CHOP, insurance, lymphoma, ManyPets, Milo, Miniature Dachshund, remission, scan
November 2023
Pupdate
Sadly Milo’s cancer is back, which I touched on the the relapse diary post. At least we caught it early with a scan, so he’s not actually been ill; and like the last time the chemo doesn’t seem to be denting his enthusiasm. The end of the month also marked the anniversary of when he first got ill, which seems like a significant milestone to get past.
It’s got cold now (with some light snow even), so the boys are back to wearing their fleeces on walks.
Whisky
I typically go for a tasting, or just drinks with friends, at the Scotch Malt Whisky Society every 6 weeks or so. But this month brought more than the usual visits, and the first one was pretty special, a tasting of Royal Brackla.
It’s not a distillery I’d heard of before, which is hardly surprising as their output is fairly small, and most of it has previously gone into blends. The tasting opened with their 18yo, which was delightful, and probably the best of what we had (even though older and more expensive varieties followed).
Much was made of the award winning streak of Dewar’s master blender Stephanie Macleod who oversees production at Royal Bracka, and that was enough to get me to buy a few Dewar’s bottles during the Black Friday sales. Their 12yo is a brilliant example of what a blended whisky should be.
The Bacardi/Dewar’s/Royal Brackla team brought along some ‘new make’ from the distillery, which is spirit straight from the stills before any ageing in wood. At this stage it’s emphatically not whisky as it hasn’t had the mandatory 3 years minimum maturation. I thought it tasted a little like grappa. It definitely wasn’t just tasteless pure ethanol and water, and it was interesting to find those same flavours in a 12yo society bottling from the distillery that was also featured in the tasting.
Many thanks to India from the Barcardi team for her excellent overview and willingness to answer questions.
Solar Diary
This November was much the same as the last overall.
New home lab server savings
It’s looking like the new ‘server’ consumes about 22.5kWh per month less than the old one, which will save me around £75/yr giving a payback (just on energy) of around 3y. Not bad considering how much more speedy and capable it is versus the previous kit.
Beating Beat Saber – Quest 3
Apparently I wasn’t alone having controller tracking issues with my Quest 3. After reading my October post a friend sent me a link to The Verge: “Meta is investigating Quest 3 issues after controller tracking complaints”. Thankfully it wasn’t long before reports emerged that Meta Just Fixed the Quest 3’s Big Controller-Tracking Problem. I’m not sure that it’s entirely fixed, as I’ve had one more episode of a saber wandering off on its own, but it certainly seems better.
My new corrective lenses also arrived, so I’ve been able to play without the bother of getting the headset on whilst wearing glasses :)
I’m not blown away by the Quest 3. It even seems to stutter in the same place on levels that could be problematic on the Quest 1; though maybe that’s a game issue rather than hardware.
Although I’m still not impressed with the head band, at least I didn’t buy the fancy expensive one with an additional battery as reports are now emerging of charging issues.
Filed under: monthly_update | Leave a Comment
Tags: whisky, pupdate, solar, Quest 3, Royal Brackla, Dewar's
AI MacGuffin
I’m increasingly hearing AI talked about as a MacGuffin… It could magically solve this thorny and expensive problem that’s been intractable for years. We all just need to geek a bit harder, buy (or rent) more GPUs and scrape together some better training data…
In this context AI cannot fail, it can only be failed, by insufficient investment/commitment/belief.
Filed under: technology | 1 Comment
Tags: AI, hype, MacGuffin
Milo’s scan at the end of last week showed some areas of concern, so it was back to the vets for a biopsy, which confirmed the reoccurrence of Mott cells. The lymphoma is sadly back, and so we’ve restarted chemotherapy with CHOP protocol again.
We’d maybe got to a point where we were holding out hope that he’d stay well. But that isn’t in the nature of the disease.
On the other hand he had no external signs of trouble, so the scans have let us catch things before he got unwell.
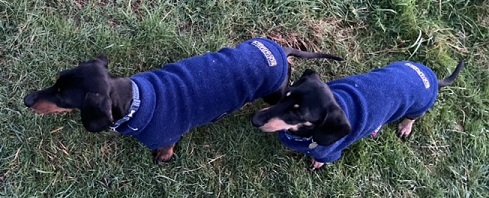
So… it’s back to weekly vet visits after what seems like a huge (and much appreciated break), and crossing fingers that the neutrophils aren’t too low.
Emotionally things are a lot less difficult this time. This wasn’t unexpected. We know what happens next. Our local vets are up to speed already. Of course there’s still sadness, but we can cross our fingers and hope for some more fun months with him, which might just turn to years if we’re really lucky.
Past parts:
Filed under: MiloCancerDiary | 1 Comment
Tags: biopsy, cancer, chemotherapy, CHOP, Milo, Miniature Dachshund, Mott cell, scan
October 2023
Pupdate
The first half of October continued the fine weather we enjoyed through September, so there were more chances for long walks through the local fields and woods.
NYC
My trip report for a visit to New York got a little too long for this post, so check out the standalone post if you’re interested in airlines flying to/from Gatwick and a bag that’s designed specifically to fit within silly new carry on limits.
Home lab upgrade
I’ve been doing a bunch of stuff with OpenWRT lately, which has meant lots of waiting around for builds to finish. I thought maybe it was time for some faster (and more energy efficient) hardware underneath the VMs in my home lab, and it wasn’t long before I was down the rabbit hole of TinyMiniMicro. When I emerged I found myself a Lenovo M720q[1] on eBay with a decent spec and a decent price[2].
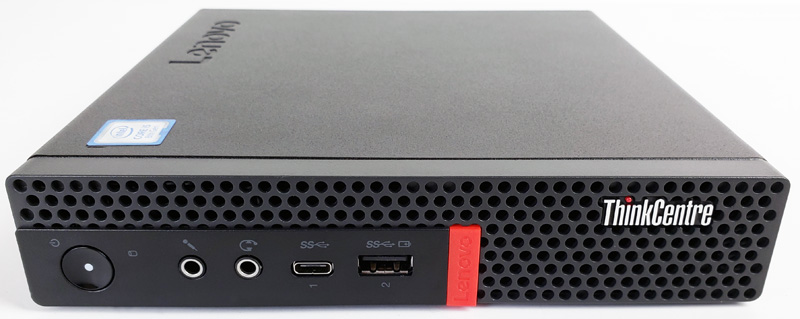
ESXi 8.0u2 installed without any snags, and when I moved the VMware datastore SSD over from the old server it was trivial to get my existing VMs running :) The only small sticking point was finding somewhere to host the 3.5″ SATA drives I had in the old server, which has been taken care of by an ORICO 5 Bay Enclosure. I’ve had to put some tape over the power switch so it starts up after a power cut[3], but apart from that it’s working, and seems like a sweet piece of kit.
Solar Diary
It might have been warm at the start of the month, but overall it was less sunny than last year.
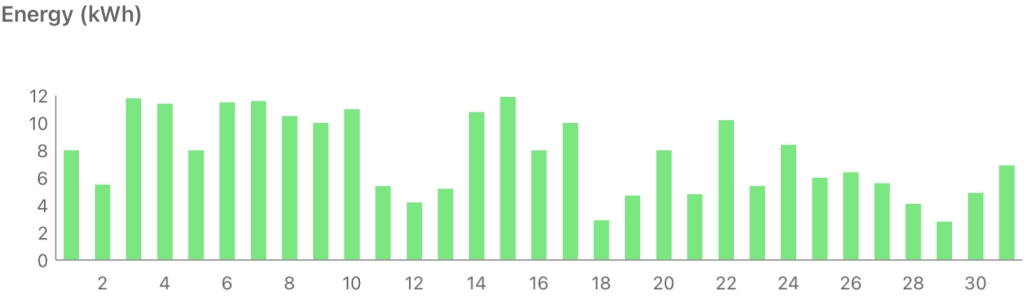
I’m starting to keep track of my iBoost readings so that I have a clear idea of electricity that’s being used generally versus what’s being used to offset gas for hot water. Hopefully that will help me identify the savings from the new home lab gear.
Beating Beat Saber – Quest 3
Having stuck with the original Oculus Quest it was time for an upgrade. I skipped the Quest 2, as when it launched it required a Facebook account. Thankfully they’ve rolled back on that one, so I ordered a Quest 3 straight after launch, and it arrived ahead of schedule.
Sadly first impressions weren’t good:
- It takes different corrective lenses, so I’ve had to order new ones, and wait weeks for them to arrive[4].
- The headband is far less comfortable than the original (and I can now feel why I maybe should have taken a look at the premium ones offered at checkout).
- Hand controller tracking fails with alarming regularity, which can really mess up a good run in the game when one of your swords suddenly drifts off.
- I can’t really tell that there are more pixels and a faster CPU. I expected things to be sharper and more fluid, and they’re just not.
Hopefully things will pick up as the platform matures. But usually it’s the v3 where companies really get it right, and Meta seems to have not been paying close enough attention to quality and user experience.
It almost goes without saying that I’ve not full combo’ed any new levels :/
Notes
[1] If I’d been really thorough in my research I’d have got an M920q, as that’s the variety that comes with Intel vPro, which provides remote keyboard/video/mouse capabilities.
[2] £225 got me an i5-8400, 16GB of RAM (now upgraded to 64GB), 1TB NVMe SSD and 256GB SATA SSD (now earmarked for my Raspberry Pi 5 when it arrives). I may upgrade the CPU to an i9-9900 if I’m ever doing stuff that stretches the existing CPU, as they seem to be readily available on Ali Express.
[3] There shouldn’t be any power cuts, as I have a UPS. But the UPS batteries had failed, and just like every other UPS I’ve ever encountered there was no indication of a problem until it just didn’t work. For now it’s on my spare UPS whilst I fix the (larger) original one.
[4] They did arrive before the end of the month, but then the battery was flat on the headset, so I’ve not been able to test them yet.
Filed under: monthly_update | Leave a Comment
Tags: Beat Saber, NYC, pupdate, Quest 3, solar, vr
New York – Oct 2023
I joined a couple of my colleagues at the ONUG Fall 2023 event so that we could talk to people about the Networking 2.0 stuff we’re building at Atsign.
For the trip to New York I was pleased to find that I could get there and back from Gatwick (and at a reasonable price).
Travel
jetBlue LGW-JFK
I remember friends in the US raving about jetBlue, but I’ve never flown with them myself. They now do transatlantic flights, and things are a bit different from other carriers:
- They’re using a narrow body Airbus 321LR rather than the wide bodied aircraft that usually ply the transatlantic routes.
- Roughly half the cabin is ‘Mint’ business class pods, which look very nice. With the rest being economy, including a few rows of “Even More Space” extra legroom seats.
- They offer (very reasonable) one way fares, which makes it possible to avoid the usual silly prices unless you stay over Saturday rigmarole from the traditional carriers.
- Flights from Gatwick, which for me avoids a 1-1.5hr £100 taxi ride (that takes me right past Gatwick).
My flight was somewhat marred by one of the two toilets in the ‘core experience’ (economy class) being out of action, but apart from that it was pretty decent. I liked that they had free WiFi, though it was a bit patchy, and blocked SSH got in the way of some work stuff I’d wanted to do. Lunch was decent, though sadly they follow the typical US pattern of having no soft drinks without caffeine and/or high fructose corn syrup :(
We arrived a little early, and the tiny international arrivals part of their terminal got me on my way pretty promptly.
Sonder Henri on 24
Finding a hotel near the venue at a reasonable price was the usual NYC challenge, and having had a good experience with Sonder in Chicago I thought I’d try them again. Henri on 24 was within walking distance of the event, and also just a block away from one of my favourite haunts – Barcade Chelsea.
My little studio had everything I needed for a few days, and it was great to be able to use wired Ethernet during my All Day DevOps presentation (which I’d signed up for when I still expected to be in the UK).
Norse JFK-LGW
Norse are a new airline with a familiar feel. It’s the rebadged Norwegian 787s from their transatlantic fleet, flying the same routes, with the same execs running the show, and some of the same silly rules :(
- 10kg limit on carry on (they even impose this on ‘Premium’ tickets now, which used to allow a much more sensible 15kg).
- No online check-in – you have to go to the desk (so that they can check carry on is within limits).
- No TSA Pre, as they’re not yet integrated with the programme.
But… they beat jetBlue on price for my return leg, and they also fly to Gatwick. I took some comfort in knowing what to expect from previous experiences flying Norwegian.
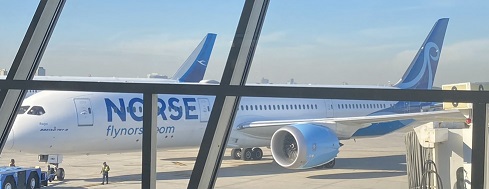
They fly from JFK Terminal 7, which is BAs old terminal, and perhaps even more grim now than it was in the BA days. The only lounge is the Aer Lingus one, which has stopped doing day passes. Though it was possible to find somewhere comfortable to sit, and the free WiFi worked well enough.
Check-in was a bit of a zoo (even in the premium line) with lots of time consuming friction around carry-on and “personal item” size and weight limits. Though when it was my turn the process was quick and efficient enough (it was just slow for everybody else that showed up with too much stuff in the wrong sort of bags). Boarding seemed pretty smooth though, and the onboard experience was exactly what I expected from previous Norwegian flights.
They got me to Gatwick ahead of schedule, and I was home within an hour of wheels down, which was great, as I’d be lucky to be getting into a taxi at that point on a Heathrow flight (only to then contend with the M25 at rush hour). Despite previous reservations about using Norwegian for business travel, I’d probably go with Norse again based on this experience. For one thing they seem to have sorted out punctuality :)
Bags
Threading the needle of the different rules for the two airlines meant some changes from my usual US loadout.
jetBlue’s dimensions of 22x14x9″ for a carry on are too small for my 22″ roller (even though I’m pretty sure it would have easily fit on its side)[1]. And I’ve been caught out before by Norwegian’s Norse’s ridiculous 10kg limit. So the roller stayed at home, and I used the Lowe Alpine Pioneer 26 I bought in August for the far East trip as my carry on. But it’s not big enough for everything I wanted to take, and for the Norse leg I needed something I could put the heavy (but small) stuff in to keep below the weight limit.
Enter the Aerolite 40x30x15cm, a bag that’s specifically designed for the size limit for a ‘personal item’ that’s been imposed by most European carriers post Covid; a limit that’s also used by Norse.
There’s room for the gadget bag I keep handy when flying, my cables bag, my power bank, my iPad, my toiletries, my hydroflask and my laptop; which is all the heavier stuff, and also all the things that need pulling out when going through security. So it’s a pretty handy way of organising everything. I could see it being sufficient for a business daytrip, or even a weekend if I was travelling tech light.
Patagonia make a bag they call the MLC for Maximum Legal Carry-on. This is maybe an MLP, for Maximum Legal Personal-item. For comparison the MLC is 45 litres, whilst the MLP is 18.
Trtl Travel ‘Pillow’
I came across Trtl last year, and put one on my Christmas list. This was my first time trying it (as it was my first overnight flight that wasn’t business class). It’s not a pillow at all, but rather a plastic neck brace covered in some soft fabric. It worked really well, and I managed a few hours of decent sleep. Given that it packed into my hand luggage easily I’ll be happy to give it room on future trips.
Note
[1] It was good to see the carry on rules being well enforced by jetBlue during boarding, which meant we didn’t end up with any silliness of people with bags far from their seats, or having to gate check stuff. There was even room in the overheads for some of those ‘personal items’ to go up after takeoff.
Filed under: travel, review | Leave a Comment
Tags: jetBlue, Norse, Sonder, Aerolite, bags, carry on, personal item, Trtl
September 2023
Pupdate
September brought some fine weather, so the boys have been able to enjoy more extra long walks at the weekend :)
Milo scans
Milo’s cancer care has settled into monthly scans, covered in the latest diary entry. TL;DR – he’s doing OK.
Cars
I’ve driven Volvo’s almost all my life, and I’m presently on my third[1] a 2010 model XC60 that I bought in 2013. It’s been a great car – comfortable, practical, nice to drive. Sadly it let us down at the end of last month. The starter would turn over the engine, but it just wouldn’t fire up. The problem was a burnt out fuel pump connector[2], which meant an expensive and prolonged visit to the garage.
While the Volvo was away that meant my daughter’s Mini was being used for most trips. Pretty handy for the Ikea run, especially given the expansion of London’s Ultra Low Emissions Zone (ULEZ). After one trip out Rachel came back home saying “I think I’d quite like a Mini, but one of the bigger ones”.
So we’re now the proud owners of a Countryman Cooper S. I’ve not had much of a chance to drive it yet, but it seems nice (and fun).
Horsted Place
Last month brought a whole series of posts about the 2nd honeymoon trip for our 25th wedding anniversary, but because of work schedules we had to do that before the actual event. To celebrate the proper date we found a local hotel that looked like it would be nice – Horsted Place.
It was absolutely lovely. We were treated to an upgrade to their master suite, which seemed like somewhere that a well heeled gentleman could spend an entire season. The weather was hot and sunny, so rather than just having pre dinner drinks in the lovely garden we stayed out there for dinner too. It was a shame to have to leave (after a delicious breakfast), but sadly work beckoned.
KTM Motocross Experience
Last summer my daughter was showing some interest in motorcycling. I think she wants my ’95 CB1000 when I’m done with it. I went looking for a way that she could try out biking, without just driving around a car park on compulsory basic training (CBT). What I found was the KTM Motocross Experience, where one of the venues, Golden Barn, is only a short distance from home. After eventually finding some dates that weren’t booked solid (or clashing with the second honeymoon) we managed to go just before she had to head back to University.
It was a roasting hot day, but I’d take that over rain and mud. There was a good build up from basic riding safety around the oval, to a reduced circuit, to the full circuit, and every bit of it was great fun (but also intensely hard physically and mentally).
Here’s one of my latter laps:
Steve Hogarth
I’ve been a huge fan of Marillion since back when Fish was the lead singer, so when the chance to see their present lead playing ‘H Natural’ came up I didn’t hesitate to buy tickets.
It was a wonderful gig with a fantastic mix of well loved Marillion tracks, Steve’s solo material, and covers.
Solar Diary
Although September was (mostly) sunny, it really shows the difference that the seasons make when looking back to June.
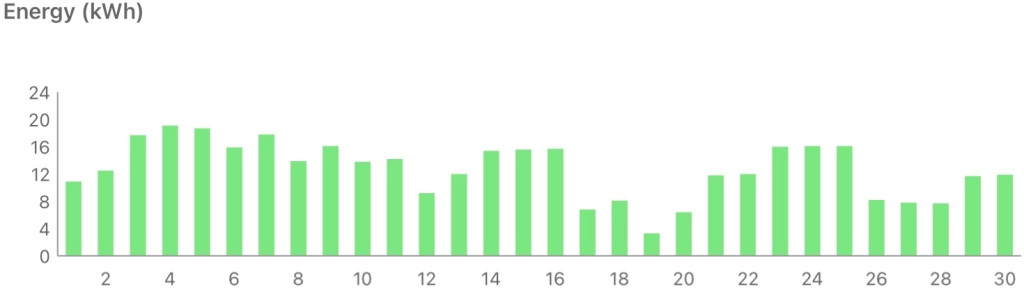
SEG paid
Last month I mentioned that I was expecting £59.16 for Smart Export Guarantee, and that got paid pretty swiftly :)
Notes
[1] I previously had a 340DL, which had been my Dad’s. He was going to trade it in for a new Seat, but I persuaded him to do a swap for the junker Renault 12 I’d bought shortly after my 18th birthday. Later on we got a V40 (when a family friend got caught drink driving in it and realised they’d never get their license back). It was a sheep in wolf’s clothing, looking like the racy T4 model, but actually a regular 1.6 SE.
[2] Apparently not uncommon on this model.
Filed under: monthly_update | Leave a Comment
Ever since I started signing GitHub commits with SSH keys I’ve made sure to use git v2.35 or later. Unfortunately Google Cloud Shell comes with a rather crusty old version of git (as part of the fact that it’s still based on Debian 11 ‘Bullseye’).
$ git --version
git version 2.30.2
Just copying over a more recent git binary doesn’t work. There’s also little point trying to update it using regular methods, as changes outside of the home directory get overwritten. We need to install into the home directory, so it’s necessary to build and install it locally from source[1].
First create a .local/bin directory and add it to the PATH[2]:
mkdir -p .local/bin
PATH="$HOME/.local/bin:$PATH"
Then make a place to download the source, cd into it, clone the source, and cd into it:
mkdir -p git/github.com/git
cd git/github.com/git/
git clone https://github.com/git/git.git
cd git
For HTTPS URLs to work we need libcurl4-openssl-dev for the build:
sudo apt install libcurl4-openssl-dev
If a build was done now, it would be of whatever is in the master branch, which might be unstable, so better to checkout the latest stable release, which is v2.42.0 at the time of writing:
git checkout tags/v2.42.0 -b v2.42.0
Then configure, build and install:
make configure
./configure --prefix=${HOME}/.local
make install
The new git should now be on the PATH:
$ which git
/home/chris/.local/bin/git
And it should be much newer than 2.30.2:
$ git --version
git version 2.42.0
Notes
[1] Well… you could cheat and grab a precompiled tarball from the v2.42.0 release on my fork.
[2] There should be a section like this in ~/.profile, but that might be missing from really old instances of Cloud Shell:
# set PATH so it includes user's private bin if it exists
if [ -d "$HOME/.local/bin" ] ; then
PATH="$HOME/.local/bin:$PATH"
fi
Filed under: cloud, howto | Leave a Comment
Tags: cloud, git, github, google, shell